This is Your Future Health Care on AI
Columbia researchers and clinicians are studying how artificial intelligence can translate to better patient care
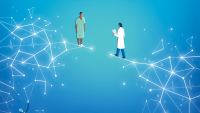
By Sarah C.P. Williams, Portraits by Jorg Meyer, Illustration by Davide Bonazzi
Several years ago, cardiologist Pierre Elias, MD, was working overnight in a cardiac intensive care unit when he was called by another hospital’s emergency room about a man complaining of shortness of breath and chest tightness. Three months prior, he had presented with the same symptoms. A quick EKG displaying the heart’s electrical activity as squiggles and lines showed nothing abnormal, and the emergency room had sent the man home with the good news that he was not having a heart attack.
On the night Dr. Elias answered the call, the man had returned to the emergency room, but this time he could barely breathe and doctors quickly put him on a ventilator. An ultrasound revealed that the valves in his heart were severely diseased and could no longer open and shut correctly—a problem with the heart’s structure rather than its electrical activity.
“If he had been diagnosed with valvular disease the first time he came in, he could have had a procedure to fix it,” says Dr. Elias. “But by the time he came back, he was already in multi-organ failure.”
The man died, but his story has stuck with Dr. Elias, assistant professor of cardiology and biomedical informatics and medical director for artificial intelligence at NewYork-Presbyterian. Patients like that man inspired Dr. Elias and his colleagues to turn to artificial intelligence to analyze routine EKGs and chest X-rays to predict whether patients are at a high risk of structural heart defects and need further tests.
“Even though I’ve looked at 15,000 electrocardiograms in the last decade, there are still pieces of information, such as structural disease, that I can’t interpret from them,” says Dr. Elias. “It turns out that an AI model can do it with a much higher degree of accuracy than me or any other cardiologist.”
The program that Dr. Elias developed to predict structural heart disease is one of hundreds of AI-based technologies that are being built and tested at Columbia and NewYork-Presbyterian with the goal of helping clinicians provide patients with better, more personalized care.
“We are not far away at all from computers being able to analyze patients’ medical records, make treatment plans, correct errors, and help clinicians in many other ways,” says Jason Adelman, MD, associate dean for quality and patient safety at VP&S. “It’s very promising.”
Thousands of tools are in various stages of development and study around the world, and the FDA has approved nearly 900 AI- enabled medical devices as of May 2024. For people at the helm of medical technology, this imminent paradigm shift means that now is the time to prepare.
“Before anything is used at the bedside, we need to make sure that it is safely and equitably advancing care,” says Peter Fleischut, MD, group senior vice president and chief information and transformation officer at NewYork-Presbyterian. “We need to go through an extensive review of each new technology.”
Columbia and NYP, he says, are poised to be leaders in this field. The diversity of patients in New York City, the close integration of researchers and clinicians, and the willingness of the health system’s leadership to embrace innovation provide an ideal testing bed not only for new AI technology itself, but also for how to seamlessly assess and integrate AI into clinical care. “We’re really positioned well to be the go-to place to evaluate, accelerate, and utilize these technologies,” says Dr. Fleischut.
Bringing Data Together
While training to become a neurocritical care doctor, Soojin Park, MD, remembers wishing that she could assemble all the data on her patients into one platform. Most of Dr. Park’s patients have severe brain and spinal cord injuries; the only way she can gauge their progress is through a plethora of monitors that measure things like brain function, breathing, blood pressure, and heart rate. But as recently as the early 2000s, few of these monitors were connected to each other—or to any external systems.
“It was difficult for clinicians who were trying to monitor dozens of patients at once, because there were all these standalone devices and you could only view their data by walking over to the bedside,” says Dr. Park, associate professor of neurology (in biomedical informatics).
Dr. Park also suspected that if she could mine all the data from the neuro ICU, she might be able to predict which patients were most likely to have complications, such as strokes.
“The problem with this idea is that it was putting the cart before the horse,” says Dr. Park. “I knew there was valuable information in that data but I couldn’t get it. It was a big engineering hurdle.”
Dr. Park spent the next decade working with some of the first methods to pull data, in real time, off the bedside machines in the ICU to one interface. Columbia was one of the first medical systems to implement that remote monitoring technology, which quickly made it easier for doctors to understand if a patient’s status changed, even from another room. It also paved the way for Dr. Park to apply AI methods to the vast amounts of data coming from each patient’s bedside.
“There can be really subtle changes to a patient’s physiology that a human can’t pick up on but an AI program can detect,” says Dr. Park.
Over the course of several years, Dr. Park and her colleagues developed AI tools that could predict—hours before nurses and doctors typically notice anything awry—when patients in the neurocritical ICU were likely to develop complications. Today, the program is running in the background at Columbia/NYP, analyzing real-time data on hundreds of patients.
“We’re at the stage now where we know that this AI model can work, but we need to figure out how to translate it to clinical use,” says Dr. Park.
Figuring that out involves comparing decisions that clinicians make for patients with advice that might be given by the AI model. That can reveal how and when AI could have changed patient outcomes—for better or worse.
Dr. Park’s experience, she says, underscores just how critical the underlying devices and systems in a hospital are to allowing the integration of new technologies. It took close collaboration with IT analytics teams at Columbia and NYP for her group to be able to collect, store, and analyze neuro ICU data.
“It can be tricky and expensive for hospitals to save all this data on patients, so they need to be on board if a researcher or clinician wants to use it,” she says. “NYP had the foresight to allow us to collect the data we needed and that made a big difference.”
Gaining Trust in AI
But even when a tool is shown to work, a larger challenge can be convincing clinicians that it is worth using and that it can be trusted. For more than 15 years, Herb Chase, MD, has lectured Columbia medical students on biomedical informatics and medical AI, and he says his goal is to persuade young doctors to keep an open mind about integrating the tools into their clinical practice.
“I want them to know enough that they don’t automatically reject AI tools because of things they’ve seen in the media,” says Dr. Chase, professor of clinical medicine (in biomedical informatics). “Physicians want to know exactly how new tools work, but with AI, it may be inexplainable, which can make physicians hesitant.”
Dr. Elias, for instance, tested 15 cardiologists at Columbia and NYP on their ability to diagnose structural heart disease using EKGs—both with and without the AI tool he developed to predict such diseases. Without the AI tool, the doctors were 64% accurate at diagnosing structural heart disease (it is not surprising that this number is so low, Dr. Elias says, since EKGs are not typically used to diagnose structural problems). But even with the AI model, the doctors were only slightly better, at 68% accurate.
“It turned out they didn’t trust the model, so they often ignored it,” says Dr. Elias. “These top-notch cardiologists would see that the AI model was suggesting structural heart disease but they couldn’t see any of the signs themselves, so they’d go with their own gut and say there was no disease.”
It is not a problem unique to AI, Dr. Elias says. It takes time for doctors to learn to trust any new blood test or medical scan. One of the ways to hasten this trust is for technology developers to work closely with doctors and nurses as they begin planning their tools, to ensure that the information they are providing will be presented in a way that is helpful.
It is a mindset that Sarah Rossetti, PhD, associate professor of biomedical informatics and nursing and former critical care nurse, uses in her research. For more than a decade, Dr. Rossetti, with colleague Kenrick Cato, has used AI to analyze how and when nurses log information on patients in acute care units and the ICU; their nursing surveillance behavior can signal their expert-driven insight on how patients are doing, even before their vital signs or lab values change.
“When a nurse is more concerned about a patient’s status they’re going to be checking on them much more frequently and documenting patient data more often,” says Dr. Rossetti. But in the past, it was hard to quantify nursing surveillance or convey to other clinicians why a nurse was so worried about one patient if the vital signs were still normal. To bridge this gap, Dr. Rossetti developed CONCERN (Communicating Narrative Concerns Entered by RNs), an AI-based tool that tracks the timing and frequency of nursing notes and other nursing assessments and interventions entered by nurses on acute and critical care units. CONCERN flags patients as low, medium, or high risk for having poor outcomes based on the data.
“Right off the bat, we knew that training clinicians so they understood this model and could very easily use it was essential to making it valuable,” says Dr. Rossetti. She and her colleagues worked closely with the Columbia and NYP IT teams and with inpatient nurses and doctors to determine the best way to make the information accessible and simple. The end result is a color-coded system (patients are green, yellow, or red) that appears on the existing medical record system.
“We’re using nursing data to give clinicians simple, straight-forward information right on their login screen so they can quickly prioritize patients and escalate care early enough to prevent adverse outcomes,” says Dr. Rossetti.
A large study of CONCERN’s use at Columbia and another large health system shows that the tool identified patients’ deterioration risk up to 42 hours earlier than other early warning systems and significantly decreased in-hospital mortality risk by 35.6%, sepsis risk by 7.5%, and length of stay by 11.2%.
Personalizing Medicine
Ultimately, AI has the potential to equalize and personalize medicine. By flagging rare conditions that are tricky for doctors to diagnose and suggesting different treatments for different patients, AI puts patients closer to the same footing—whoever their doctor is, whatever their race, and whatever insurance plan they have.
“It’s inconceivable that a human can master the entire opus of medical diagnoses and perfectly diagnose every patient on their first try,” says Dr. Chase. “But AI can come much closer to that.”
Despina Kontos, PhD, professor of radiology and vice chair for AI and data science research in the Department of Radiology, says one of the reasons that Columbia is such a prime place to test AI technologies is the diversity of its patient population. An AI tool developed in a small hospital that sees mostly wealthy, white patients may not be as effective for patients of other socioeconomic or ethnic backgrounds, and AI researchers are increasingly aware of the biases that computer programs can harbor.
“You need to be sure that any tool works equally well across ethnicities and one of the things that fueled my decision to come to Columbia was the diversity and richness of the population we have here,” says Dr. Kontos. “We’re very well positioned to derive metrics on new AI tools and how well they really work.”
Dr. Kontos has focused her research on how AI models can extract new information from mammograms. She has shown that even when someone is not diagnosed with breast cancer, certain features of the mammogram such as breast density can indicate likelihood for developing cancer in the future to suggest more frequent screenings. Technologies like the ones she has developed are being used by doctors around the country to generate breast cancer risk scores for women.
Previously, different radiologists may have given the same mammograms different breast density ratings, but an AI tool helps standardize these numbers. Now Dr. Kontos and her colleagues want to develop similar screening tools for other types of cancer, including ovarian and lung cancers.
AI also can guide treatment of cancer after it is diagnosed. Typically, when people with cancer are scheduled to receive radiation therapy to shrink their tumors, they receive a CT scan about a week before so doctors can visualize the tumor and plan exactly how much radiation must be delivered and where. But on the day of the treatment, things might look different.
“We need to compare the patient’s body on the day they come in for radiation compared to the day they got their initial CT scan,” explains Mich Price, PhD, associate professor of radiation oncology. “A tumor might have grown or shrunk, or maybe someone just drank a big glass of water, moving some of their anatomy around.”
Those changes mean that doctors must quickly recalculate how to aim their radiation. It’s usually a rough guess and means that small areas of a tumor can be missed, or radiation might be directed into healthy tissue.
Dr. Price has led the implementation of an AI-powered “adaptive radiation” system that can analyze a patient’s CT images on the day the patient arrives to receive radiation therapy and come up with a new plan, both more quickly and more accurately.
“The system can say ‘OK, the tumor moved or changed by exactly this much,’ and then in four or five minutes it can update the planned treatment to consider these changes. It does this without the patient having to get off the machine or receive an updated CT scan, additional work that normally takes us a about a week to complete,” says Dr. Price.
The AI program, which is now in use at Columbia/NYP, keeps patients from waiting, saves time for clinicians, and makes it more likely that the desired amounts of radiation are given to the right parts of a tumor. But it still requires clinicians to ultimately sign off on the plan and supervise the radiation therapy.
“AI is not replacing us,” Dr. Price says. “AI is a tool that is enabling us to provide high quality care to more patients more efficiently.”
Harkening in a New Era
Nearly every area of medicine and hospital administration may soon be transformed by new AI technologies. At the new Center for Patient Safety Science, Benjamin Ranard, MD, an intensivist and deputy director of the center, is evaluating two different AI models to predict when patients might develop sepsis. Dr. Chase is using AI to study interactions between drugs, guiding which medications people should be prescribed when they are taking dozens of pills. Other Columbia researchers have used AI to diagnose dementia from data on older people’s driving patterns, to predict preterm births, and to detect eye diseases. Hospital administrators are eyeing new AI tools that can write clinical notes and streamline billing and admission processes.
But many of these tools have only been tested in research settings, and their integration into large hospital systems will take time—and intensive planning.
“You can generate a new tool that works, but when you put it into the clinic, there are suddenly all these new questions: How does it affect a clinician’s workflow? Does it get reimbursed? Who has liability if the tool finds something? How do you communicate the findings to a patient?” says Dr. Kontos.
There are also basic questions about whether the tool is useful in the first place. “If you have an AI model that can predict with complete accuracy when someone is about to die, that would be a very impressive tool on paper,” says Dr. Adelman. “But if it’s only providing this information when doctors are doing chest compressions on a dying patient, it doesn’t really add anything to help patients. At Columbia, we’re trying hard to make sure that any AI model we use is translating to improved care.”
At Columbia and NYP, clinicians, researchers, hospital administrators, and IT experts are collaborating closely to address these challenges and create a smooth approval and implementation pathway for effective, safe AI tools to advance patient care. More than 140 AI tools are in various stages of study and use at NYP, says Dr. Fleischut, and large teams are being assembled across the system to study and prioritize new AI-based technologies. Many tools are running in the background—collecting and analyzing data but not influencing decision-making —so they can be closely monitored.
“I think it’s important for big medical centers like Columbia to pave the way and show that we can be forward thinking while still being thoughtful about our patients and their privacy,” says Dr. Kontos.
A common concern about AI technologies in medicine is that they might replace doctors or remove the human element from medical treatment. But most researchers who work with AI say those concerns are overblown—and they are the same hesitations that arise with every new technology.
“There was a time when physicians listened to patients’ hearts by placing their ear to the patient’s chest. Physicians did not want to use stethoscopes because their use would create a barrier between the patient and physician,” says Dr. Chase. “But these are important tools that can expand a doctor’s wisdom.”
Like a stethoscope, an AI program can give doctors new information that helps them make the best decisions possible for their patients.
“Good, smart human doctors and nurses make errors all the time,” says Dr. Adelman. “I imagine a world where AI can tap them on the shoulder, figuratively speaking, and say ‘Are you sure about that? You might want to consider an EKG. You might want to reconsider that medication.’ That kind of assistance could make our hospitals better and safer.”